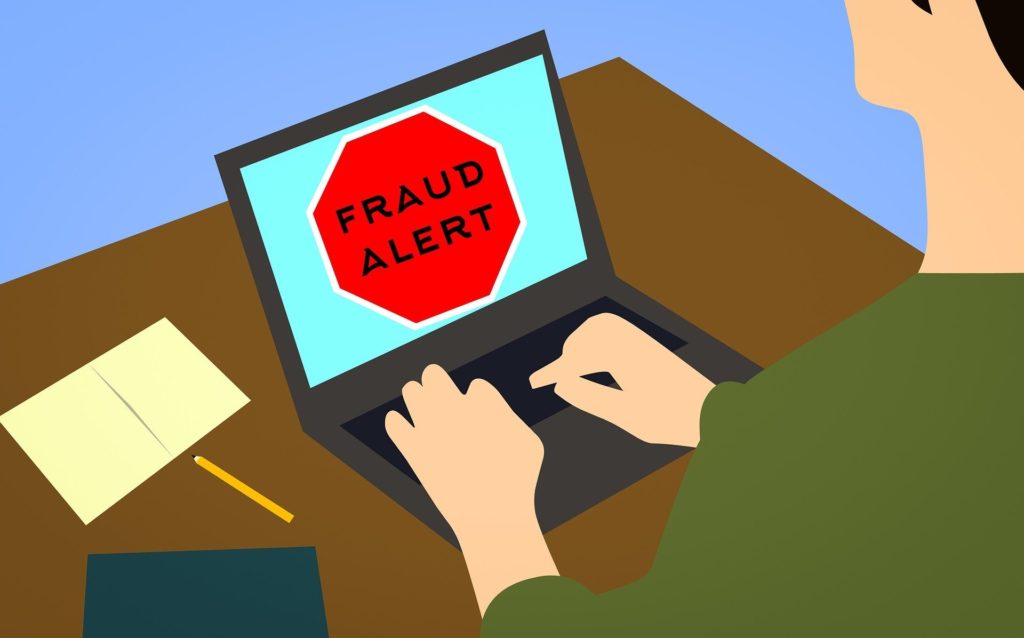
Theft Detection & Alert Mechanisms :
Energy theft is regarded as one of the most expensive kind of thefts. They often happen with a direct tap into a smart grid’s distribution system. From a data standpoint, this anomalous activity stands out from usual operations. Systems can be devised in order to detect theft in real-time and notify the authorities.
How do we do it?
Anomaly detection is based on classification algorithms, which study data on all types of energy distribution activities & tag the anomalous ones. In real-time, when an activity that falls into the anomalous category, alerts can be triggered and theft can be stopped.
Preventive Maintenance of Equipment :
With the help of Internet of Things (IoT) enabled machineries, it is possible to collect vital stats of machines at fixed time intervals. This data is utilized to extract the maximum possible work out of them, while halting them for preventive maintenance at the appropriate time. This minimizes the down time due to failures, while maximizing the useful effort extraction from the machines.
How is it done?
There are two approaches to this problem. A classification model can tell us the likelihood of the failure of a machine a given time frame. When the likelihood goes above a threshold value, maintenance is performed.
Alternatively, a deep learning model can predict the Remaining Useful Life (RUL) of a machinery at any given time, based on its vital statistics.
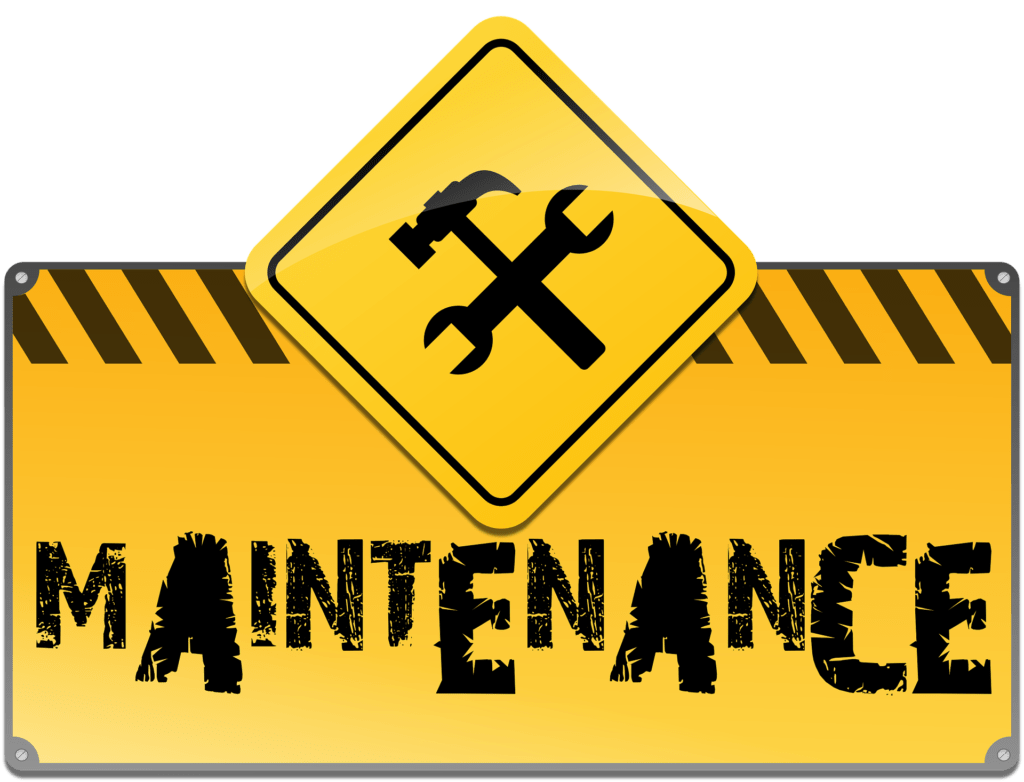
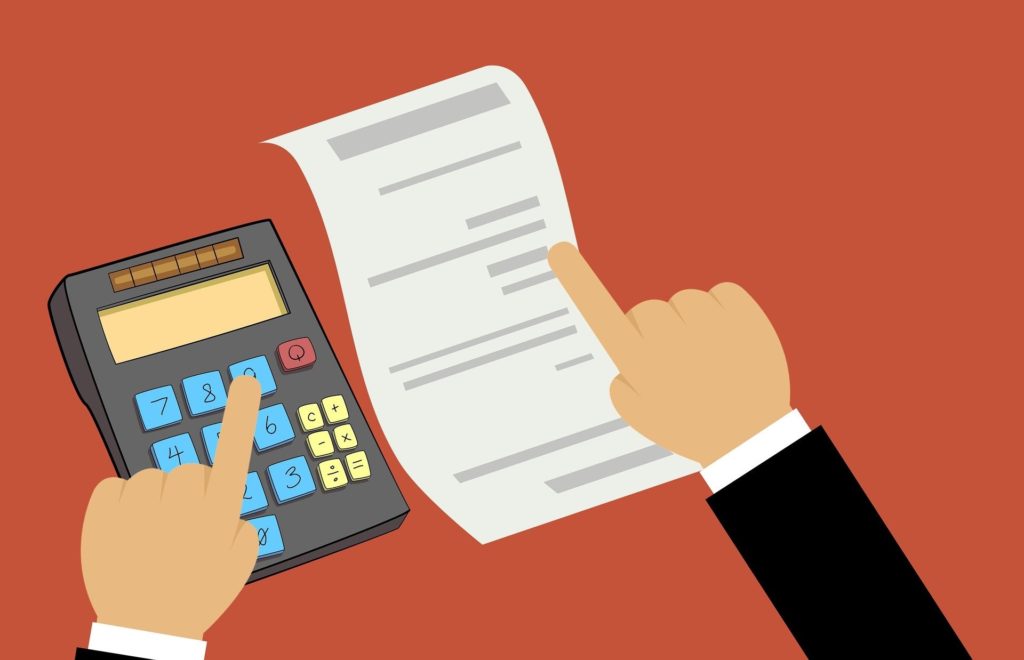
Demand Response Management :
Successful energy management strategies rely on perfecting the balance between demand and supply. Both high and low demand rates can hurt growth and efficiency. It is essential to track demand in real-time and possibly predict demand too, in order to stay ahead of it.
How to go about it?
Real-time management applications monitor energy use, identify and track energy peaks and adjust the flow of energy as per the demand at any given time. With enough data collected in this manner, it is possible to explore it and look for patterns and consistencies in it. This can be used to make predictions on energy demand in a given time frame.
Outage detection and prediction :
Blackouts can be prevented with an automatic protection system in place, that is capable of predicting influence of weather conditions on the power grid, detecting the possibility of outages based on smart meter events. With the right KPIs and their threshold values, outage can well be predicted and prevented.
How do we do it?
By leveraging historic data on power outage and carefully analysing their root causes, predictive machine learning algorithms can be trained to look for these patterns in real-time data. The application of the smart energy outage systems allows providing accurate real-time outage statuses to improve general customer experience and satisfaction.
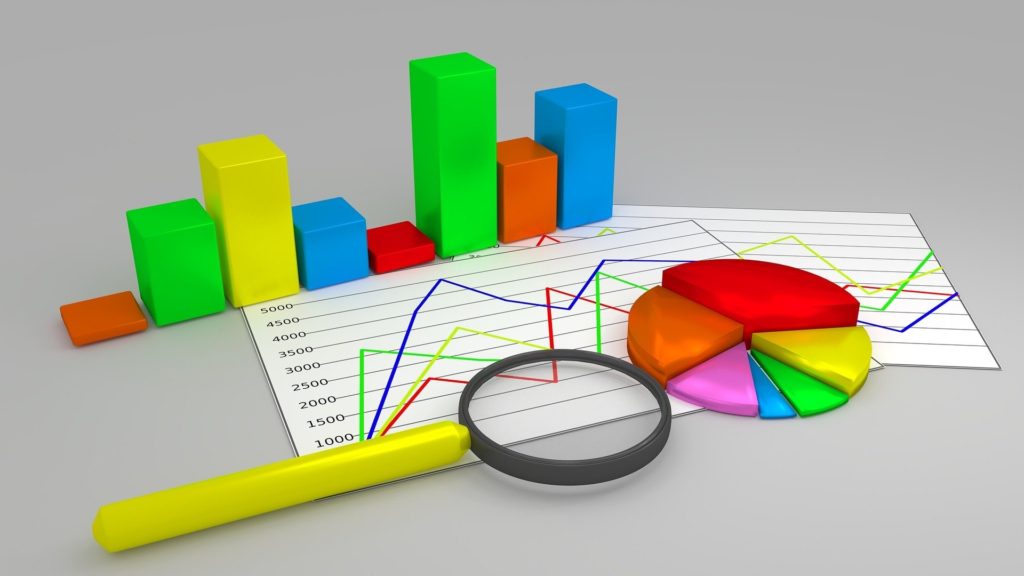