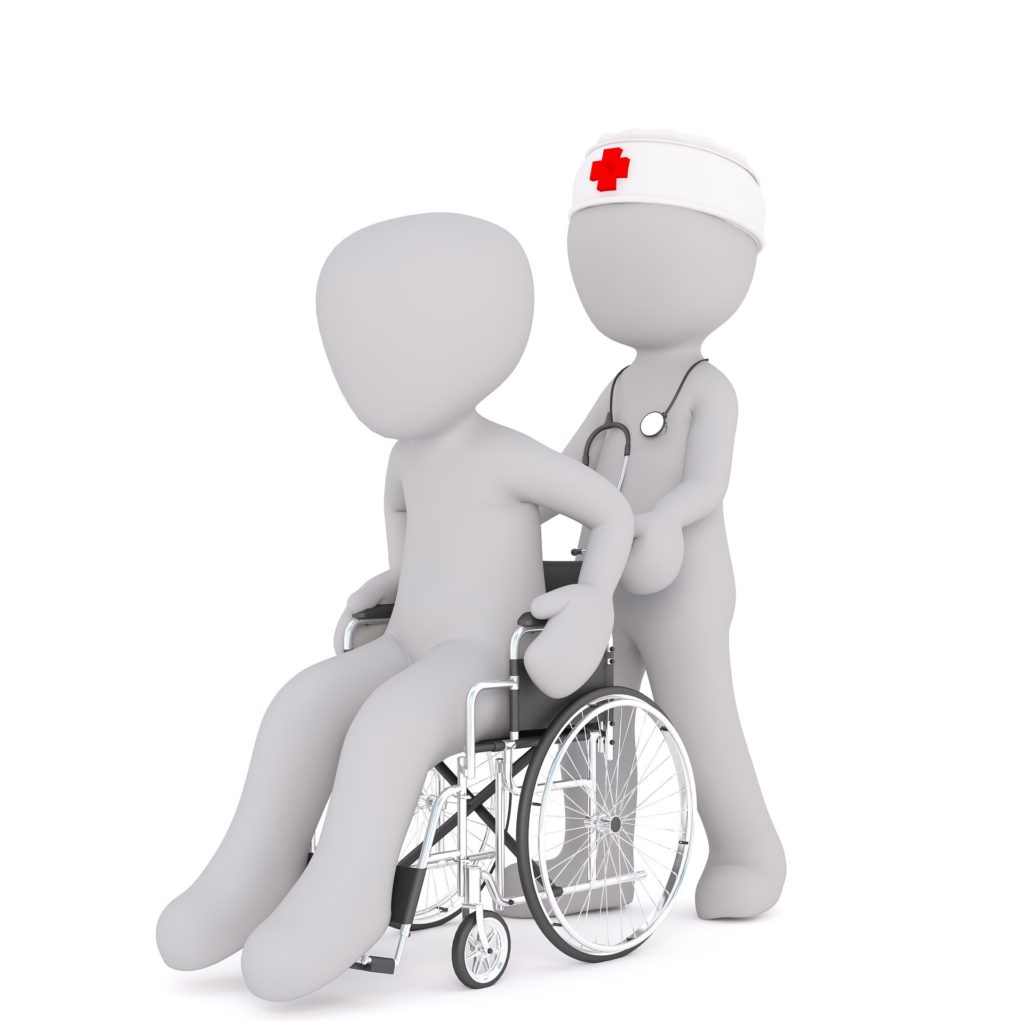
Patient Inflow Prediction :
The inflow of patients is seasonal, cyclical and often predictable, if historical data is leveraged. Being prepared for a sudden increase in inflow of patients or toning down on operational expenditure during a predicted low activity period can save lots of resources and efforts.
How do we do it?
Regression based Machine Learning algorithms can be trained using the historic data available on patient inflow. They can extrapolate the inflow on a given time frame, whose accuracy of prediction can be gauged by RMSE metrics.
Doctor performance analysis :
Unbiased physician performance analytics provides the tools for physicians, providers and payers to identify overuse, underuse and misuse in physician performance that impacts medical costs and patient outcomes.
How is it achieved?
Our BI solutions provide comprehensive reporting and access to key productivity, physician cost, revenue cycle and practice financial data at every level of the organization. Physician data from multiple sources can be integrated into one common backend, which can be ingested into our data visualization and analytics tools, in order to have real-time access to physician metrics.
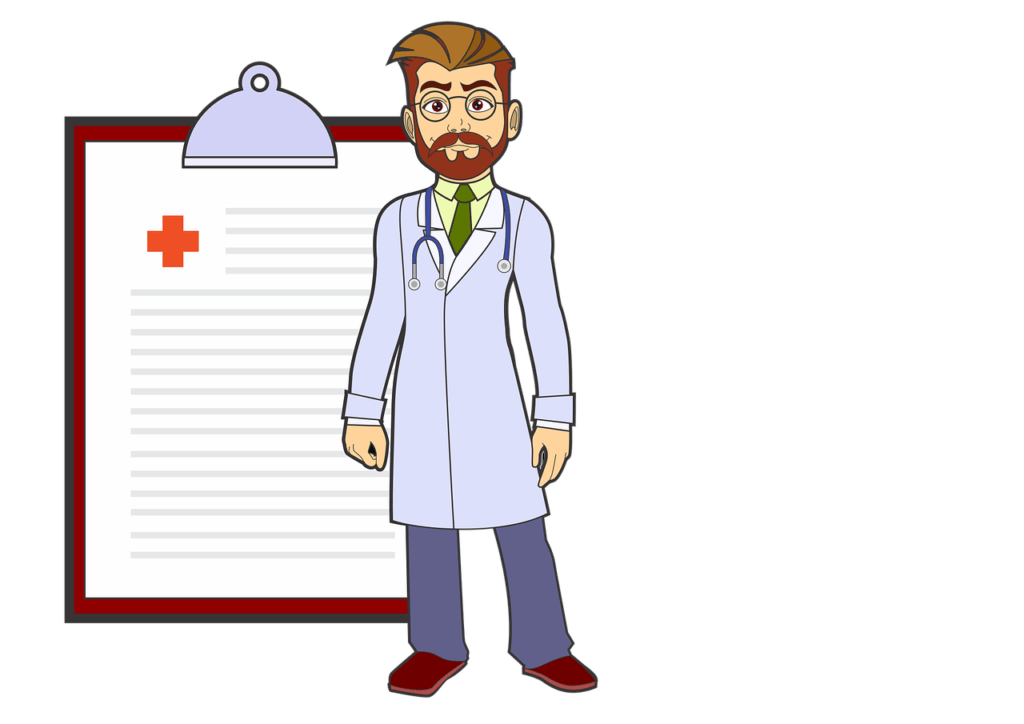
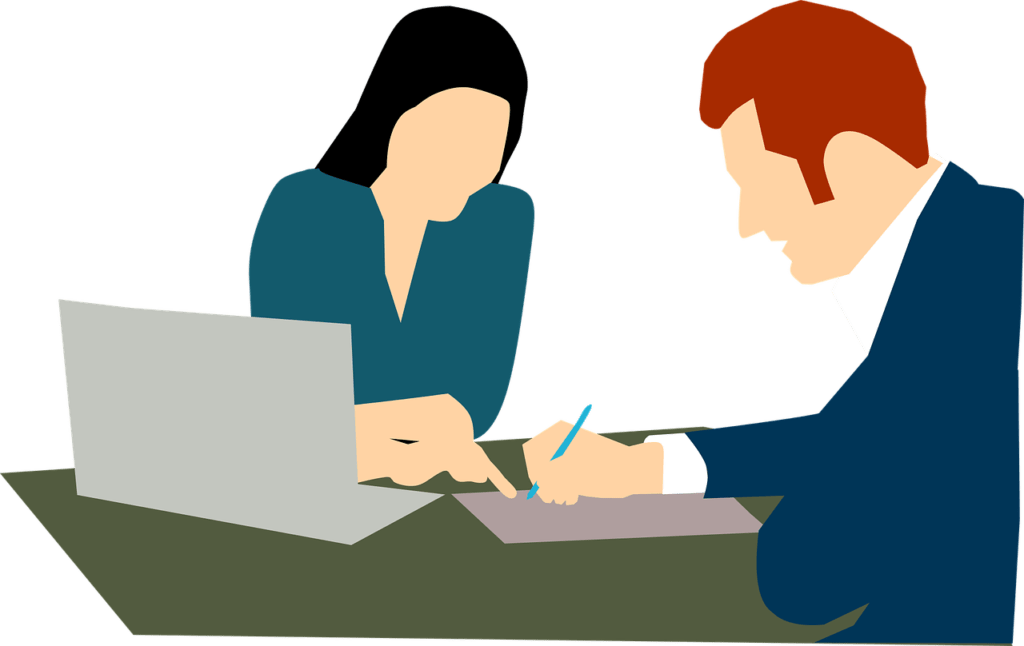
Insurance Analytics :
In order to sustainably serve patients, while avoiding possible losses, hospitals need to make the right partnerships with carefully thought out terms. This means having the means to quantify the risk associated with each patient signing up for the insurance services.
How do we achieve it?
Using the data available on claims made, patient history, treatment and expenditure records, machine learning algorithms can provide the probability of the likeliness that a particular patient would file for claims, based on a few predefined factors. Classification and logistic regression algorithms provide the best solutions.
Revenue Cycle Management :
There are inefficiencies through the different stages of the revenue management cycle which include pre-registration, communication to the payer, claims submission, admission and discharge documentation, and query response time and payable tracking and follow-ups.
How can we be of help?
Predicting payer remittance dates to manage organizational cash flow, identifying potential claim denials before they happen, pinpointing revenue cycle management inefficiencies and breakdowns in the early stages, leveraging machine learning to predict changes in payer-specific claims adjudication rules, decreasing dependency on IT resources for accessing necessary information are some of the approaches that can be leverage for effective revenue cycle management.
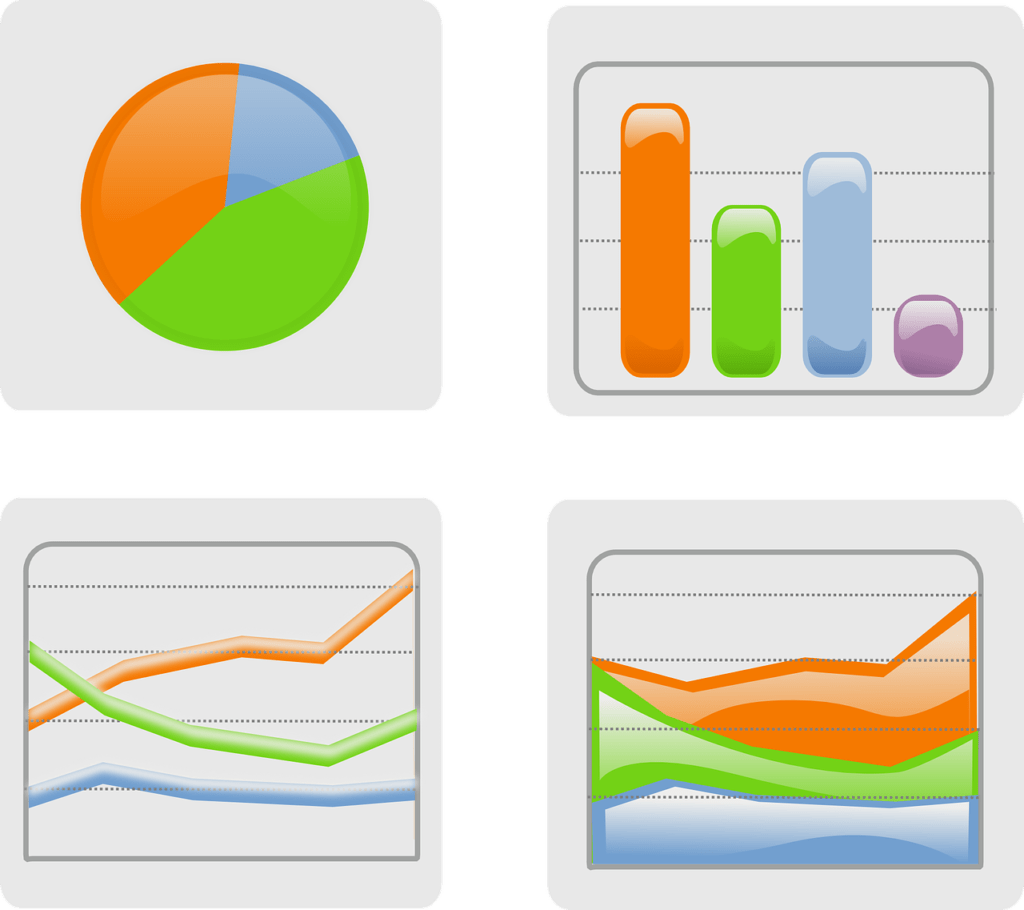
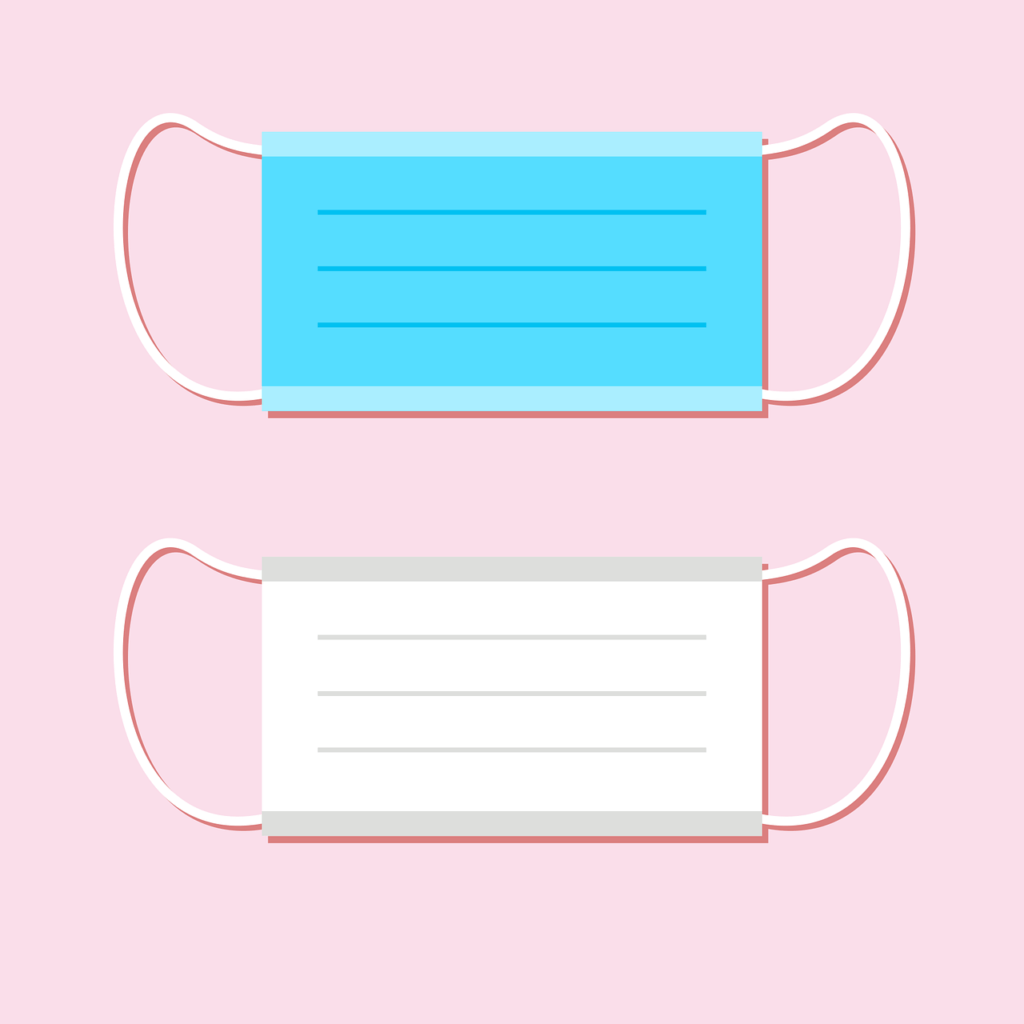
Readmission Reduction :
Health systems can achieve significant reduction in readmissions by fully integrating predictive analytics strategies into their workflows. Applicable predictive models will consider the entire patient readmission journey, as well input from the whole care team and the patient.
How is it achieved?
Predictive analytics approaches with the right feature selection related to patient demographic information, diagnosis and treatment history can help with individual risk assessment of patient readmission. Randomized logistic regression can be used to evaluate the relevance of features. Rare events are eliminated during the data preparation stage to improve the accuracy.
Hospital Acquired Infection Analysis :
Health care-associated infections (HCAIs) are those infections that patients acquire while receiving health care. These increase healthcare costs, and are a major safety concern for both patients and healthcare professionals.
How do we go about it?
EHR data with geospatial mapping tools provides a uniquely useful visual exploration of how multi-drug resistant organisms (MDROs) spread between patients. Images of the hospital floor plan can be imported into Tableau. Tracing patients along their journey through the physical environment helps the health system understand patterns in different infections.
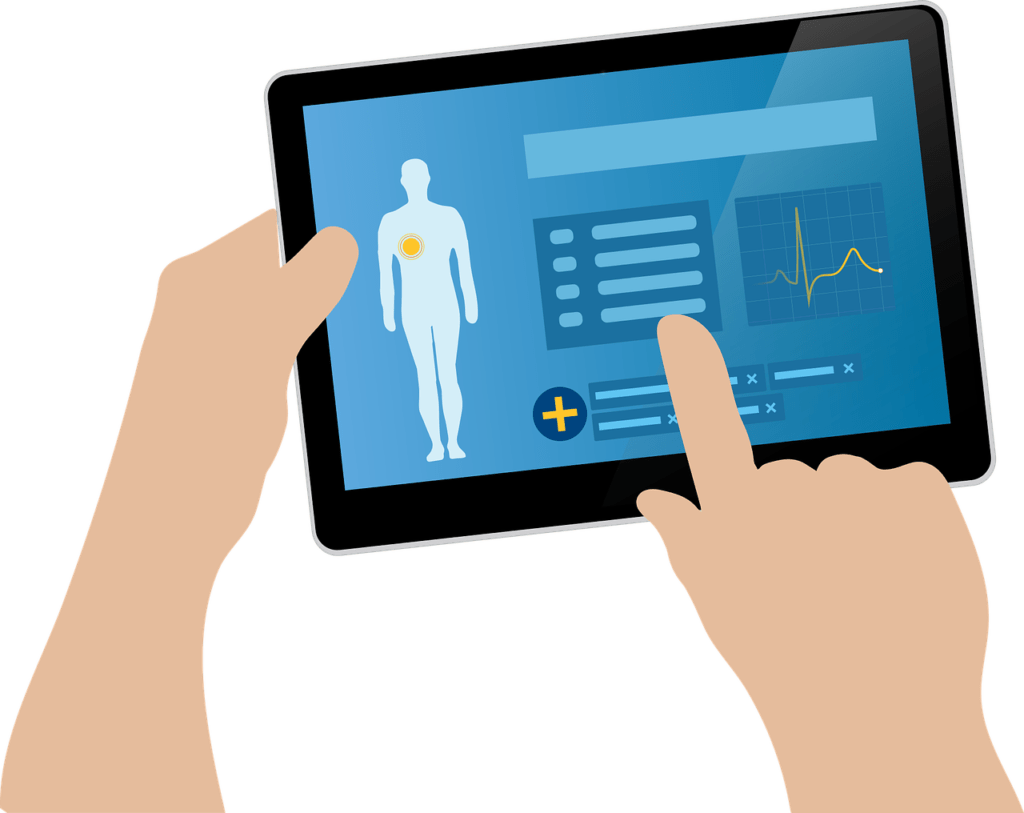